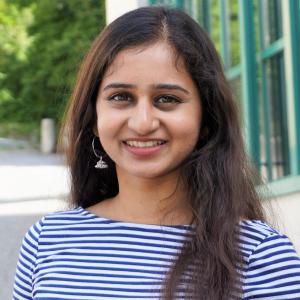
Srilaya Bhavaraju
MIT EECS | Quick Undergraduate Research and Innovation Scholar
Using Locality-Sensitive Hashing on ECG Waveform Data
2017–2018
Electrical Engineering and Computer Science
- AI and Machine Learning
Una-May O'Reilly
Erik Hemberg
Locality sensitive hashing (LSH) addresses the nearest-neighbor search problem of machine learning. The essence of LSH involves hashing similar input items to the same buckets with high probability and without the need for model-based learning. This project aims to explore the applications of LSH in health care by efficiently finding patients with physiological waveforms similar to a reference waveform. A given similarity set can then be exploited for future or diagnostic extrapolations to the patient of reference. Through this project, I will investigate LSH in prediction problems such as EEG, ECG, and ABP, and explore extensions of LSH, such as whether hashing families can be combined or whether different hashing families should be used for different predictions.
As a student whose interests lie at the intersection of technology and medicine, I find that this project falls perfectly into that intersection. I have had experience applying machine learning techniques to social media data and am excited about exploring machine learning applications within health care as well as gaining more insight into the problems affecting health care.