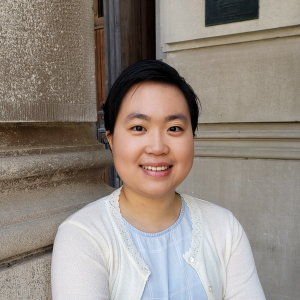
Song Eun Kim
MIT EECS | Analog Devices Undergraduate Research and Innovation Scholar
Using E(3)-Equivariant Features for Autoregressive Transition Metal Complex Generation
2023-2024
Electrical Engineering and Computer Science
- Electronic, Magnetic, Optical and Quantum Materials and Devices
Tess E. Smidt
Molecular generative models can help navigate the enormous space of chemical compounds for applications in medicine, energy storage, and industrial catalysis. We develop an E(3)-equivariant autoregressive model to generate transition metal complexes, aiming to predict the ligand positions for a given central metal atom. Euclidean symmetry-equivariant neural networks (E(3)NNs) like this one are particularly well suited to handling the data types used to describe atomic structures because they account for the Euclidean symmetries found in 3D space: 3D rotation, translation, and inversion. This is part of our ongoing project to predict metal-organic chalcogenide assemblies (MOChAs), crystals containing low-dimensional transition metal chalcogenide structures scaffolded by organic ligands.
The intersection of machine learning and physics is fascinating to me, and I look forward to more deeply exploring this area in general, and molecular generative models in particular, over the course of this SuperUROP. As part of Prof. Smidts group, I have had the opportunity to make progress on a generative model for small molecules, and I am excited to continue developing this model for more targeted forms of material design.