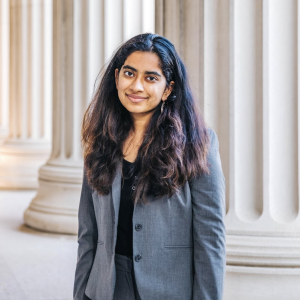
Sadhana Lolla
MIT EECS | Gerstle Undergraduate Research and Innovation Scholar
Learning Generalizable Control Policies for Soft Robots from Self-Supervised Morphological Features
2022–2023
Electrical Engineering and Computer Science
- Artificial Intelligence and Machine Learning
Daniela L. Rus
Current methods of automating the design and control process of soft robots, such as co-design, are computationally expensive. One more efficient approach to automating this process is learning a morphology-agnostic controller using expert collected data, wherein the controller acts based on the intrinsic sensory readings and extrinsic morphology. Deep-learning based approaches to learning such a controller are fully supervised and are fundamentally limited by highly imbalanced training datasets. This work aims to develop a more robust control policy for soft robots that leverages self-supervised learning in order to better learn feature representations from unlabeled data and adaptively guide the design optimization landscape, thereby enabling the policy to generalize to new morphologies.
Through this SuperUROP project, I want to become more involved in the application of deep learning algorithms to robotics. I hope to apply knowledge from previous machine learning classes and research to an exciting new area of study.