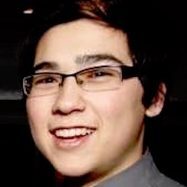
Ryan Chung
MIT EECS Lal Undergraduate Research and Innovation Scholar
Automated Protein Sequence Annotation
2016–2017
Electrical Engineering and Computer Science
- Machine Learning
Bonnie A. Berger
Automated Sequence Annotation using Recurrent Neural Networks
Identifying the structure of proteins is key to understanding their underlying functions. The three-dimensional structure depends on complex interactions between the amino acids and the cellular environment and is therefore a difficult task. This task can be relaxed by only considering local structures within the protein termed the protein’s secondary structure. Various machine-learning approaches have been used to predict protein secondary structure such as SVMs CNNs and HMMs with some success but were unable to capture long-range dependencies. In this study we solve the problem of annotating the secondary structure class of each amino acid directly from the sequence. Our preliminary classification accuracy with stacked LSTMs on the TS1199/TR4590 dataset1 is over 80%.
My fascination with neural networks and machine learning began with my high school research and grew when I applied these tools to biological systems in my UROP last summer. I’m excited to use these skills to tackle a difficult but high-impact problem. Through this project I hope to develop as a researcher and presenter.