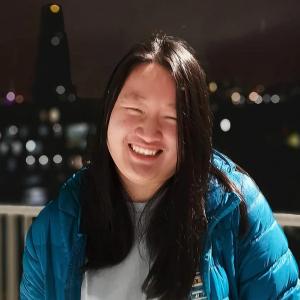
Rawisara Lohanimit
MIT EECS | CS+HASS Undergraduate Research and Innovation Scholar
Fairness in Natural Language Generation
2022–2023
Electrical Engineering and Computer Science
- Natural Language and Speech Processing
Lalana S. Kagal
Natural language generation (NLG) is a technology that enables the generation of human-readable language for various tasks, including virtual assistants, chatbots, machine translators, summarizers, and composers. These applications interact directly with people to generate content they specialize in across many domains, such as health, education, or customer service [1]. Although these applications can effectively produce natural text, they can also introduce unfavorable societal biases that reduce their effectiveness or harm marginalized groups. An educational chatbot may deter users of a particular ethnicity from using it, for instance, if it responds negatively to that ethnicity. Due to the lack of input from that racial or ethnic group, the chatbot will become more prejudiced as it continues to learn. Bias NLG not only harms marginalized groups but also has a negative impact on the general population. The model that produces bias response can potentially create a negative representation of a particular demographic. Users will receive inaccurate information as a result, which could lead to misrepresentations or the spread of stereotypes [1]. Since NLG directly interacts with a lot of users, it is crucial to address the issue of misinformation by developing a fair NLG.
We propose to apply a box-of-the-box controlling model on top of the pre-trained model. Inspired by GeDi and DExperts, we aim to control the generated text through a lightweight bias model that anyone can train on. By creating a bias model, we will use that probabilities output to modify the original probability distribution and eventually lessen societal biases such as gender, race, religion, and profession. After that, we intend to assess the bias in our models in comparison to the original models using various datasets and matrices.
I am participating in SuperUROP because I want to gain more research experience. Understanding how machine learning learns, including biases, is one thing I am curious about. My previous UROP and internship help me to learn about computer vision and I want to explore a new area though this project. I am excited to contribute something meaningful to the machine learning community and see how much I will grow at the end of it.