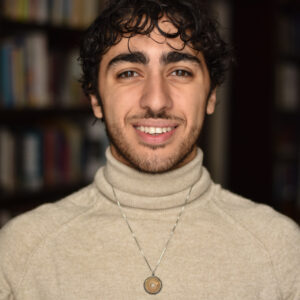
Omar Dahleh
MIT Tang Family FinTech Undergraduate Research and Innovation Scholar
PrivateNLG: A System for Synthetic Data Generation for Mixed Data Types
2023–2024
Electrical Engineering and Computer Science
- Privacy in Machine Learning
Lalana S. Kagal
In a world of increasingly ubiquitous machine learning tools and in particular large language models, the question of privacy in LLMs has become pressing and prevalent. Large language models (LLMs) are predicated on the usage of large swaths of training data. Privacy attacks that leak units of data which are often private and confidential have been more common in the advent of publicly available LLM tools such as ChatGPT and others. My research, a collaboration with Liberty Mutual Insurance, on their claims dataset, introduces a system for synthetic data generation for structured (tabular) and unstructured (free text) data that achieves both a high level of privacy and immunity to attacks, while maintaining the original attributes that make the data effective for generating LLMs.