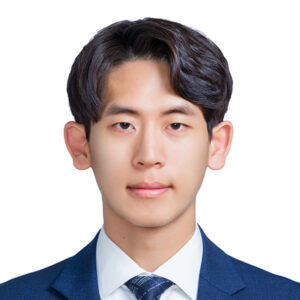
Kiwhan Song
MIT EECS | Nadar Foundation Undergraduate Research and Innovation Scholar
Diffusion Forcing 2: Flexible Video Generative Modeling with History Guidance
2024–2025
Electrical Engineering and Computer Science
- Graphics and Vision
Vincent Sitzmann
In this project, we develop the next version of Diffusion Forcing, a general sequence diffusion model with unique capabilities. Through several technical improvements such as latent diffusion, our goal is to showcase its enhanced performance across multiple domains such as video, natural language processing, and planning. We aim to highlight its unique features, particularly compositionality, which are challenging for baseline models, including standard diffusion models. Additionally, we will investigate the application of Diffusion Forcing in video-related tasks, including text-to-video and novel view synthesis.
Through SuperUROP, I aim to deepen my research in generative models and computer vision, collaborating closely with our talented group. With a background in machine learning research, I am excited to not only demonstrate our framework’s capabilities and publish our findings, but also to provide the research community with impactful, practical open-source codes and models.