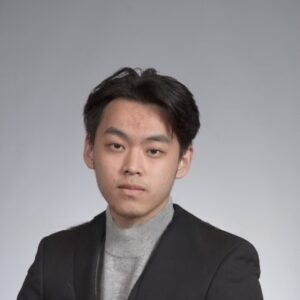
James Liu
MIT EECS | Nadar Foundation Undergraduate Research and Innovation Scholar
Training-Free Activation Sparsity in Large Language Models
2024–2025
Electrical Engineering and Computer Science
- AI and Machine Learning
Yoon Kim
Activation sparsity can enable practical inference speedups in large language models (LLMs) by reducing the compute and memory-movement required for matrix multiplications during the forward pass. However, existing methods face limitations that inhibit widespread adoption. Some approaches are tailored towards older models with ReLU-based sparsity, while others require extensive continued pre-training on up to hundreds of billions of tokens. Existing training-free approaches do not obtain substantial model-wide sparsity (around 25%). We aim to develop a method that obtains high training-free model-wide activation sparsity, and translate this to end-to-end wall-clock speedup.
I’m participating in SuperUROP to gain more exposure to the intersection of Efficient Machine Learning with Large Language Models (LLMs) — a field that I have previous exposure to, but am looking to immerse myself more deeply in.