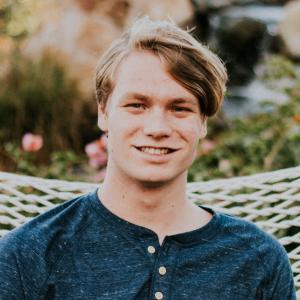
Griffin Leonard
MIT EECS Undergraduate Research and Innovation Scholar
ML Classifiers for Argumentation Teaching Strategies
2021–2022
EECS
- CS+HASS
Justin Reich
Eliciting Learner Knowledge (ELK) is a simulation to help preservice teachers (PSTs) learn and practice understanding what their students know. ELK is part of the Online Practice Suite (OPS), a set of tools being developed to give preservice teachers PSTs practice teaching skills in increasingly complex simulations. Last Spring, I developed a codebook to identify PSTs use of questioning strategies (to elicit students claims, evidence, and reasoning about math and science concepts) in ELK. The goal of my SuperUROP is to create text classifiers for these strategies that can then be implemented into the ELK platform to provide PSTs with real-time feedback on their performance and can assist researchers in analyzing large scale datasets from ELK.
Through this SuperUROP I want to gain practical experience with machine learning classifiers. This SuperUROP will allow me to apply the skills I have learned in AI and machine learning courses to a real project, allow me to build on my previous UROP work, and create a direct impact on preservice teachers by improving their learning tools. Furthering my experience with machine learning and research will be useful skills for my future career.