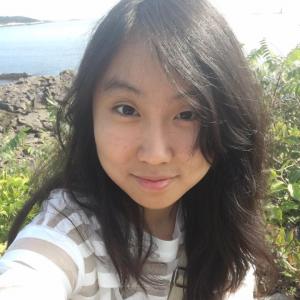
Cynthia Tianqing Liu
MIT EECS | Advanced Micro Devices Undergraduate Research and Innovation Scholar
Alignment as an Indicator for Two Distinct Training Regimes
2018–2019
EECS
- Artificial Intelligence & Machine Learning
Aleksander Madry
Deep learning is quickly becoming more prevalent in both research and industry. To make use of deep learning models, we must train a model on some task. While there are many optimizers and schedulers designed to improve training results or increase convergence speed, there is little understanding as to why these methods work. To fill this gap, we hypothesize that the training process of deep nets can be separated into two distinct phases: a generalization phase and a convergence phase. To provide evidence for this hypothesis, we observe a metric called “alignment,” which measures whether our training steps are moving us towards the solution minima during training. We hope to use this metric to demonstrate that there are two distinct phases of training, and potentially suggest a new training scheduler that takes advantage of this fact.
Through this SuperUROP project, I would like to explore deep learning while applying machine learning and mathematics to real-life problems. I previously conducted research in applied machine learning and reinforcement learning. This SuperUROP project will let me expand on that experience and tackle machine learning from a more theoretical angle, while allowing me to utilize principled reasoning skills learned in classes such as 18.100B (Real Analysis) and 6.046 (Design and Analysis of Algorithms).