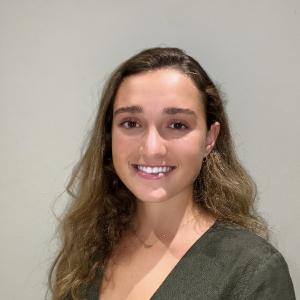
Ana Fiallo
MIT EECS | Analog Devices Undergraduate Research and Innovation Scholar
Optimizing Low-Cost Air Pollution Sensor Networks within Urban Environments
2021–2022
EECS
- Environment
David Hsu
With growing urbanization, the associated air pollution continues to be a threat to societies and a leading cause of disease, death and climate change. Without comprehensive systems of data collection, the gravity of air pollution cannot be accurately measured, thus limiting the development of mitigation tactics against this phenomenon. The optimized distribution of sensors in cities provide higher resolution data of pollutant levels, helping further reveal major causes. Leveraging linear regression of Gaussian processes and the improved prediction of mutual information, we apply London’s sensor network data and develop models to determine the optimal placement of existing sensors within this network to increase network certainty. Along the way, we investigate the areas of greatest uncertainty in the network and the relation these locations have with demographic distribution across London, thus revealing potential risks of environmental injustice and inequality through limited access to reliable air quality data.
By participating in this SuperUROP, I hope to gain extensive research experience that combines my interests in environmental sciences and urban planning, while also applying the lessons learned throughout my CS courses at MIT. My previous UROP experiences involving applied ML to environmental resiliency, along with my classes in algorithms, ML and modeling, have prepared me to tackle this SuperUROP’s environmentally-focused optimization problem.