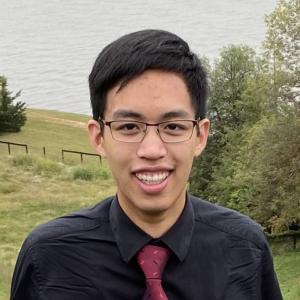
Zhening Li
MIT EECS | CS+HASS Undergraduate Research and Innovation Scholar
When Do Skills Help Reinforcement Learning? A Theoretical Analysis of Temporal Abstractions
2023-2024
Electrical Engineering and Computer Science
- AI and Machine Learning
Armando Solar Lezama
Skills are temporal abstractions that intend to improve reinforcement learning (RL) performance through hierarchical RL. Despite our intuition about the properties of an environment that make skills useful, a precise characterization has been absent. We provide the first such characterization, focusing on the utility of deterministic skills in deterministic sparse reward environments with finite action spaces. We show theoretically and empirically that RL performance gain from skills is worse in environments where solutions to states are less compressible. Other theoretical results suggest that skills benefit exploration more than they benefit learning from existing experience, and that using unexpressive skills such as macroactions may worsen RL performance. We hope our findings can guide research on automatic skill discovery and help RL practitioners better decide when and how to use skills.
My previous UROP projects helped me discover my research interests in AI for science and neurosymbolic learning. Since these projects focused on experimental evaluation of ML models and algorithms, I wanted to explore ML theory through the SuperUROP program. One of my previous UROP projects studied symbolic RL skills for neurosymbolic reasoning, which was the inspiration for my SuperUROP project on theoretically characterizing the utility of skills for RL.