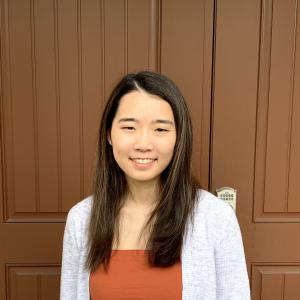
Yuria Utsumi
MIT EECS | Morais and Rosenblum Undergraduate Research and Innovation Scholar
Aligning Models for Clinical Time Series Analysis
2020–2021
EECS
- Artificial Intelligence & Machine Learning
Roger Greenwood Mark
Clinical time series is sequential data for monitoring patient health, such as vital signs and lab values. Clinicians analyze this data for applications, such as predicting in-hospital mortality or risk of heart failure for patient triaging or monitoring; however, this is a time consuming process. Latent variable models (LVMs) automate this task by inferring latent structure of observed data for analysis. For example, hidden Markov models infer states and distribution that underlie clinical time series; analyzing the clinical meaning of these states and looking at how long patients spend in each state may help us analyze a patient’s risk of mortality. However, standard inference algorithms for LVMs do not take the task of interest into consideration, so the inferred latent structure may not be best for the task at hand. This motivates our key question: how can we ensure an LVM is best aligned with a task of interest? We propose a general inference framework that integrates task information as constraints and evaluate on a mortality prediction task.
I hope to learn more about modeling for clinical applications, such as aiding clinical analysis and decision making. I am excited to see through this project end to end, learn the theoretical and applied side of machine learning in healthcare, and possibly pursue research in this area in the future.