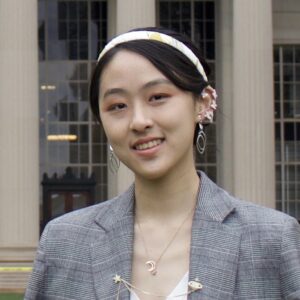
Yi (Eva) Xie
MIT EECS | Mason Undergraduate Research and Innovation Scholar
Presence of Causal Concept-based Interpretability in Network Dissection
2022–2023
Mathematics & Electrical Engineering and Computer Science
- Artificial Intelligence & Machine Learning
Aleksander Madry
Asking “why” is a fundamental aspect of understanding the world, yet measuring causal effects remains a significant challenge. Network Dissection, a concept-based interpretability method, seeks to explain why deep networks like CNNs can effectively classify images using their latent representations. However, it is unclear whether Network Dissection is confounded by the spurious correlation emerged within data or genuinely captures causal relationships. In this paper, we investigate this issue using Counterfactual ADE20K (CFX20K), a dataset of photo-realistic, paired counterfactual images. By employing Stable Diffusion to atomically remove concepts from ADE20K images without introducing confounding, we train Network Dissection and evaluate its alignment on a held-out set. Our results indicate that, unlike many other concept-based interpretability methods, Network Dissection is largely free from confounding, suggesting its reliability for out-of-distribution generalization.
SuperUROP provides me with an exciting way to conduct rigorous, meaningful research in Machine Learning. I am eager to develop modern machine learning toolkits to enable machine learning models to achieve high performance in a robust, responsible, and reliable way in the real world. I am eager to work in the Madry Lab around innovative thinkers.