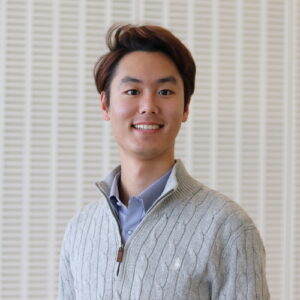
Wonjune Kang
MIT | IBM-Watson Undergraduate Research and Innovation Scholar
Certified Robustness of Neural Networks for Top-k Predictions
2019–2020
EECS
- Artificial Intelligence & Machine Learning
Luca Daniel
Deep learning models are fast becoming the method of choice for a variety of machine learning tasks in fields such as vision, language, and audio. As their prominence has increased, it has become increasingly important to examine their vulnerabilities in various adversarial settings. One line of research, called certified robustness, aims to find the largest possible perturbation to a neural network’s input that will guarantee that the model’s output will not change. For classification models, pre-existing methods are able to compute these values for top-1 predictions; that is, they can compute bounds for which a model’s top prediction will not change. However, in many applications, top-k predictions (the set of k most likely labels) are a more relevant evaluation metric. This project aims to extend these baseline methods to the top-k case by computing perturbation bounds for which the model’s original top prediction will continue to stay in its top k predictions. In addition, it will aim to extend baseline methods to other tasks such as object detection.
“Despite having shown promise in many applications, AI has yet to be universally accepted as a force for good; I believe this is largely due to difficulties in understanding the inner workings of modern deep learning methods. I am participating in SuperUROP because I would like to contribute to a structured, long-term project that works to address these issues, such as robustness, interpretability, and fairness. Through this research, I hope to gain experience and become more familiar with the work in this area.”