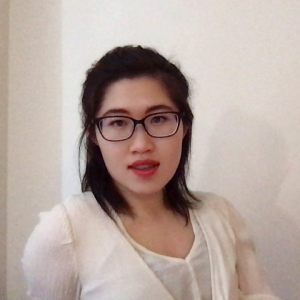
Wanyi Xiao
Undergraduate Research and Innovation Scholar
Differentially Private Federated Learning
2020–2021
EECS
- Artificial Intelligence and Machine Learning
Lalana S. Kagal
Modern AI can create models from learning data. However, data in some areas such as healthcare can be quite sensitive and therefore needs to be protected. Differential privacy is a mathematical privacy framework that captures the notion of risk when an individual is included in a dataset. This project focuses on the topic of differentially private federated learning. The objects of this project include exploring ways to generate differentially private datasets, finding better bounds for global sensitivity using these different definitions of differential privacy, adding fairness constraint to the existing federated learning model, and coming up with differentially private mechanisms for vertically partitioned data.
I want to participate in SuperUROP because I want to continue applying the knowledge about differential privacy I learned when I was doing UROP. Additionally, I want to learn how learn how to make a poster, write a research paper, and present the project in 6.UAR.