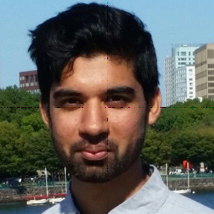
Usman Ayyaz
MIT EECS Undergraduate Research and Innovation Scholar
Making Nearest Neighbor Based Data Processing Scalable
2015–2016
Devavrat Shah
Time series data has become a modern day phenomena: from stock market data to social media information, modern day data exists as a continuous flow of information indexed by timestamps. Using these datasets to gather contextual inference and future prediction is vital. However, the massive scale of these datasets impacts latency of inference and predictions. I will be working on creating an open source platform that enables distributed time series data storage and scalable computation architecture. In particular, we are aiming at creating an architecture based on “nearest neighbor computation” accompanied with Locality Sensitive Hashing based approaches for storage purposes. In addition, we plan on integrating this platform into a time series analytics course open to the MIT community.
In the past I have worked with timeseries data, in particular Twitter data feeds to analyze viral trends. Furthermore, I was doing social media analytics with Teneo Holdings this summer which gives me a strong background for timeseries analytics. I hope to learn about research methodoligies and mathematical formulations that accompany timeseries analytics.”