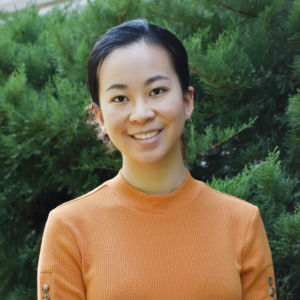
Stephanie Fu
MIT EECS | Aptiv Undergraduate Research and Innovation Scholar
Guided Feature Upsampling
2021–2022
EECS
- Computer Vision
- Machine Learning
Freeman, William T.
Previous work has shown that deep networks trained on tasks like classification or contrastive learning capture an image’ s high-level concepts. The features also have a spatial resolution, revealing where objects are through Class Activation Maps. However, these approaches are limited by the poor resolution of deep features. This project aims to solve these challenges by using guided upsampling to refine low resolution feature maps without additional supervision. Guided upsampling uses a high resolution signal, like a photo, to guide the refinement of a low-resolution signal of interest, like a depth map. This work explores whether previous successes for low dimensional signals can be applied to data with significantly greater dimensionality, as is common for deep features.
I am participating in SuperUROP because I have thoroughly enjoyed my previous UROP experiences, and I want to continue learning about both research and computer vision. I hope to also become a better researcher and gain more confidence in my skills.