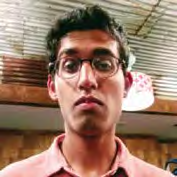
Shreyas Balaji
MIT EECS | Himawan Undergraduate Research and Innovation Scholar
Modeling Measurement Drift for Supervised Learning Applications
2019–2020
Mathematics
- Artificial Intelligence and Machine Learning
David Sontag
Measurement drift is a type of non-stationarity that refers to explicit or implicit change of encoding, where we define an implicit change of encoding as a situation when the same encoding system is used, but the physical data implied by the encoding changes over a period of time. We want to first present a series of motivating examples that show what forms measurement drift can take and how measurement drift can adversely affect predictive performance. We then wish to develop an evaluation framework for identifying and quantifying the impact of measurement drift on any particular training task. Lastly, we want to propose an algorithm to normalize for measurement drift, or construct an invariant underlying representation, so that the adverse impact on training is mitigated.
“I’ ve taken a lot of classes here at MIT theory, CS, and math and I’ m excited to finally put it all into practice. I think my project is really cool, and we’ ve already found a lot of health care applications for which this would hopefully be really useful. I hope, through this SuperUROP project, to both build practical experience training and designing models and at the same time have the opportunity to construct and test novel algorithms to improve model performance.”