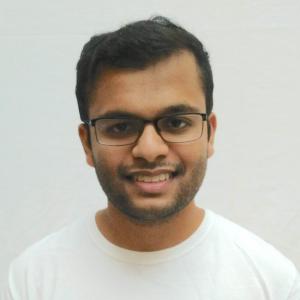
Shahul Alam
MIT EECS | Fairbairn Undergraduate Research and Innovation Scholar
Using Compressed Sensing to Improve the Efficiency of Gene Expression Data Collection
2018–2019
EECS
- Computational Biology
Caroline Uhler
Modern-day biological experimentation often necessitates extensive gene expression profiling across a variety of cell types, which in turn implies an expensive and prolonged experimental schedule. Our research aims to reduce the cost and time required to collect such gene expression data via computational methods. In particular, by leveraging the framework of compressed sensing and by taking advantage of the underlying relationships between genes, we can recover the desired gene expression data while significantly decreasing the number of empirical measurements that must be collected. Using both deep and classical machine learning techniques, we hope to optimize this scheme so that it can be employed reliably and scalably to facilitate biological experimentation in a variety of settings.
“I’ m participating in SuperUROP to hone my research skills as well as to broaden (and deepen) my understanding of both biology and computational science. I have accrued practical experience via my UROP projects in the past year as well as academic knowledge through coursework in computational biology (6.047) and deep learning (6.883), and thus I’ m excited for the opportunity to combine the two by participating in SuperUROP.”