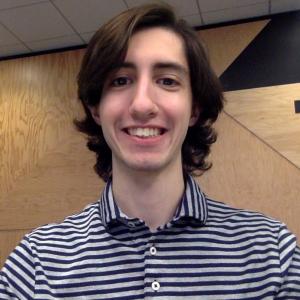
Sebastian A. Lopez-Cot
MIT AeroAstro | Lincoln Laboratory Undergraduate Research and Innovation Scholar
Improving Deep Hierarchical Reinforcement Learning Methods for Knowledge Transfer and Explainability
2018–2019
EECS & AeroAstro
- Aeronautics and Astronautics
Jonathan How
Researchers in the field of deep reinforcement learning have shown that network architectures such as deep Q-learning (DQN), deep deterministic policy gradients (DDPG), and asynchronous actor-critic agents (A3C) can produce well-trained agents to play several Atari games using only the game’s pixel array as input and the game of Go, which has an incredibly large state-space. The primary goal of this research is to understand how modeling these networks while factoring in natural language instructions might lead to more performant and explainable agents. The initial approach will be to borrow methods from hierarchical reinforcement learning to break goals into sub-goals for the agent to learn and then map an underlying language model to such a hierarchy of sub-goal execution choices.
I am participating in SuperUROP because I want to gain experience as a researcher before starting a master’ s-degree program. I have participated in the UROP program for two years and have enjoyed it very much. I am very interested in the field of deep learning and how it can be used to solve old problems in a new way. I also hope to get to better know the people in my lab through SuperUROP.