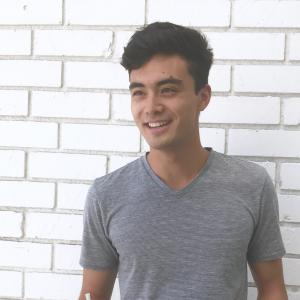
Ryan T. Prinster
Undergraduate Research and Innovation Scholar
Sequential Multitask Learning via Deep Hierarchical Reinforcement Learning
2017–2018
EECS
- Artificial Intelligence & Machine Learning
Tomaso A. Poggio
The human perceptual system can solve many vision tasks; however, current computational models are largely specialized for a single task. In this project, we aim to solve the multitask perception problem by mitigating the catastrophic forgetting observed in sequential learning of different visual problems. We will use Hierarchical reinforcement learning (RL) and elastic weight consolidation (EWC) in an effort to solve this problem, which is simplified and constrained to the Atari 2600 games.
I’m participating in SuperUROP because I want to learn to approach any new problem that my curiosity brings to me. I also think having the resources in the class will help me learn how to be more successful in a research environment. In my project, I am specifically interested in learning more about deep reinforcement learning and how it applies to solving games. Solving games and making artificial intelligence (AI) models that can compete with humans is cool.