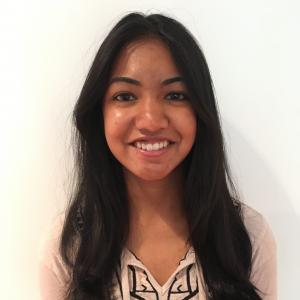
Roshni Sahoo
MIT EECS | Angle Undergraduate Research and Innovation Scholar
Developing Models Resilient to Feature-Dependent Label Noise
2019–2020
EECS
- Artificial Intelligence & Machine Learning
John V. Guttag
In real-world datasets, training labels may be inaccurate because datasets suffer from annotation error, human bias, or a noisy process for generating the labels. Label noise in training datasets leads to a decrease in classification accuracy and poses a challenge to identifying relevant features. Feature-dependent label noise is a class of label noise that has yet to be studied in great depth. Through this project, we aim to measure feature-dependent label noise in existing datasets, develop a technique for creating models that are resilient to this type of noise, and benchmark our results against existing methods for label noise reduction.
“I’m excited about SuperUROP because I want to improve the accountability of machine learning methods. I became interested in this area during my previous UROP in the Distributed Robotics Lab, where I worked on quantifying uncertainty of pose estimates. Additionally, I’ve enjoyed classes that have prepared me for this work, such as Courses 6.437 (Inference and Information) and 9.60 (Machine-Motivated Human Vision).”