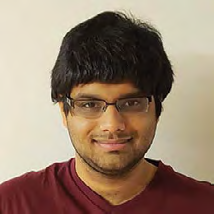
Rohan Sundar Kodialam
Undergraduate Research and Innovation Scholar
Optimal Algorithms for Ski Rental with Soft Machine-Learned Predictions
2018–2019
Physics
- Artificial Intelligence & Machine Learning
Alexander Rakhlin
The fundamental question behind most online algorithms is to determine how to deal with uncertainty of future data. In broad terms, this can be done in two ways. First, in the classical competitive analysis case, we seek an algorithm that gives a guarantee of an expected competitive ratio. The second method is to use techniques from machine learning to predict future data. If this prediction is correct, it is as if we have an offline problem instead of an online one, and we can often construct a simple and optimal solution. However, it is not immune to errors. We reconcile these two methods for a variant of the ski rental problem. In our variant, we allow the skier access to a black-box machine-learning algorithm that provides an estimate of the probability that there will be at most a threshold number of ski-days. We derive a class of optimal randomized algorithms to determine the strategy that minimizes the worst-case expected competitive ratio for the skier given a prediction from the machine learning algorithm, and analyze the performance and robustness of these algorithms.