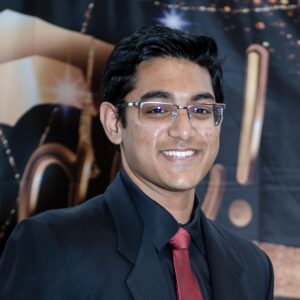
Ritik Patnaik
MIT EECS | CS+HASS Undergraduate Research and Innovation Scholar
A Gaussian-Mixture-Model-Based Approach To Classifying Vowel Place In Speech Signals
2021–2022
EECS
- CS+HASS
- Natural Language and Speech Processing
Stefanie Shattuck-Hufnagel
In recent years, speech recognition systems have dramatically improved in performance through the development of machine learning techniques. However, it is not always straightforward to interpret the mapping from the signal to the detected category. In the present work, we focus on the goal of transparency, specifying the processing steps that lead to robust modeling of vowel place using simple, descriptive Gaussian mixture models. We present a pre-processing and detection framework for vowel place, involving formant measurements, smoothing, and the GMM. This research aims to classify vowel place as a part of a larger speech recognition system. Studies were performed using ~700 vowel-consonant-vowel utterances and 8 vowel place categories based on tongue advancement, height, and root.
SuperUROP is an opportunity that I have been eyeing since Campus Preview Weekend. After participating in a couple of UROPs, I want to further develop my research acumen, especially my oral presentation and authorship skills. My fascination with speech recognition models brought me to the RLE Speech Communication Group in Spring 2021, and I am so excited to continue my work as a SuperUROP scholar.