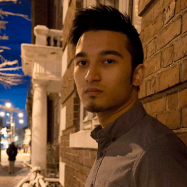
Rishad Rahman
MIT EECS | Lincoln Laboratory Undergraduate Research and Innovation Scholar
Developing a Sparse Approximation to Gaussian Curvature
2016–2017
Electrical Engineering and Computer Science
- Optics & Photonics
Justin Solomon
A simple problem that still doesn’t have a general purpose solution is approximating a surface with a planar mapping. Applications which require a grid to map onto a surface introduce some distortion and the parameterization probably has an associated metric to minimize the impact of that distortion. A disk has zero curvature everywhere so a sparse Gaussian curvature distribution will assist with the parameterization. We generate a sparse distribution is via convex optimization by looking at the transportation cost of changing the curvature distribution defined by the geodesic distances and movement of curvature between pairs of points. Another important technique which is involved in our optimization problem is that of regularization to enforce that our distribution is indeed sparse.
My SuperUROP project involves geometric data processing to analyze distortion in graphical mappings on shapes. This project will provide me with experience testing optimization methods I’ve studied experimenting with them to observe which parameters work best for the problem at hand. I love the fact that I am building upon my advisor’s research which gives me confidence in having the resources necessary to succeed.