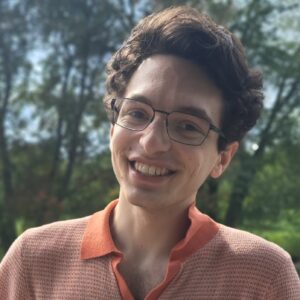
Quinn Perian
MIT EECS | Takeda Undergraduate Research and Innovation Scholar
Intersectional Debiasing for Vision-Language Models (VLMs)
2024–2025
Electrical Engineering and Computer Science
- AI and Machine Learning
Marzyeh Ghassemi
While large language models have become increasingly prevalent, these models have been repeatedly found to display concerning racial and gender bias in a wide array of contexts. Of debiasing techniques that have been developed in attempting to address these discrepancies, most do not address motivation behind choices of gender/racial categories and representations that these techniques rely on. In my project, I test whether debiased models still show improvements when using measures of bias that rely on alternative racial categorization schema or different gender/race-related features. In so doing, I aim to examine the need for more contextually-specific debiasing algorithms that are rely on race/gender features and categorizations schema most pertinent to the application at hand.
Coming from a background in critical gender and race theory, I’m excited for the opportunity to be able to bring critical theory to bear on technical questions relating to debiasing in machine learning. I hope to deepen my existing background in machine learning (and natural language processing) to better understand the state of the field and how the needs of affected, marginalized groups can be centered in debiasing techniques.