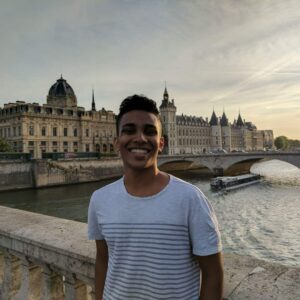
Puneeth NSK Meruva
MIT AeroAstro | Lincoln Laboratory Undergraduate Research and Innovation Scholar
End-to-End Learning of Perception Data for Autonomous Vehicles
2018–2019
EECS
- Aeronautics and Astronautics
Sertac Karaman
One common trend in autonomous vehicles is that no amount of data of real drivers is sufficient to train autonomous vehicles so that they can cover all edge-cases in addition to the more simple, common tasks. Therefore, it is critical to develop robust simulation and training engines that can produce and learn from artificial training data. In this project, we develop an end-to-end simulation and training engine based on LIDAR and camera data to train autonomous vehicles. The engine takes as an input dataset a video of a normal human-controlled drive (a base trajectory for the vehicle as a starting point) and predicts new steering-wheel angles by combining LIDAR data with the images in the trajectories, transforming the data to produce numerous artificial trajectories, and applying reinforcement learning techniques upon the new trajectories.
I have been interested in robotics and the autonomous vehicles space for more than six years. This project will allow me to acquire the knowledge necessary to excel in this field. I feel that my past internships at Uber, BMW, Microsoft Research, and the Carnegie Mellon Biorobotics Laboratory, as well as my enrollment in 6.141 (Robotics: Science and Systems) and 6.036 (Introduction to Machine Learning) have given me the necessary skills in autonomy and control to succeed in my upcoming research project.