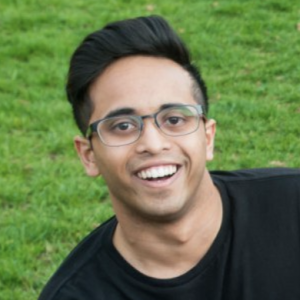
Nithin Buduma
MIT EECS | Lincoln Laboratory Undergraduate Research and Innovation Scholar
Information Sharing Among Distributed Neural Networks
2018–2019
EECS
- AI and Machine Learning
Dorothy W. Curtis
Neural networks perform well when they are built for a specific task and the set of inputs and set of outputs are well defined. However, these accomplishments are very limited in scope, and communication between different neural networks to share knowledge that will lead to the performance of more general tasks is still inadequate. We propose to utilize independent sets of neural networks while allowing knowledge transfer between each of the neural networks. The idea is based on computer network architecture. The idea will similarly allow each neural network to specialize in its own task while transferring and receiving information from other neural networks. This will allow different neural networks to be plugged in as an open platform.
“I am participating in SuperUROP because I want to apply the knowledge I have gained through taking classes covering machine learning and systems in my research. I am excited to learn how to present and communicate research more effectively.”