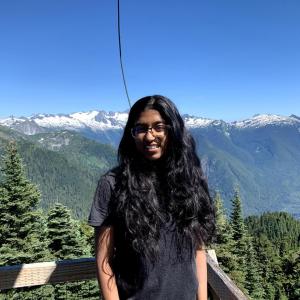
Neha Hulkund
MIT EECS | Morais and Rosenblum Undergraduate Research and Innovation Scholar
Differentially Private Methods for Domain Generalization
2021–2022
EECS
- Medical Devices
Marzyeh Ghassemi
Domain generalization is the challenge of machine learning performance on new domains. Oftentimes, poor performance on new domains can be mitigated with strong regularization techniques. Differential privacy utilizes differentially-private stochastic gradient descent (DP-SGD) to preserve the privacy of the dataset during training by clipping and adding Gaussian noise to the gradient estimate at each timestep. Both of these techniques can improve provide regularization to the model. We aim to bridge the gap between differentially private methods and domain generalization by aiming to understand the effects of gradient clipping and Gaussian noise addition to distribution shifts. We eventually plan to evaluate these techniques on the healthcare domain.
I am excited to be participating in SuperUROP to further my interests in the field of domain generalization for machine learning. I’m hoping to get a stronger theoretical background with this project and am interested in seeing its applications in healthcare domains.