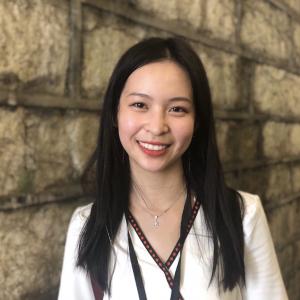
Ming Ying Yang
MIT EECS | CS+HASS Undergraduate Research and Innovation Scholar
Write it like you see it: Detectable racial differences in clinical notes lead to differential model recommendations
2021–2022
EECS
- CS+HASS
Marzyeh Ghassemi
Clinical notes are becoming an increasingly important data source for machine learning (ML) applications in healthcare. Prior research has shown that deploying ML models can perpetuate existing biases against racial minorities, as bias can be implicitly embedded in data. In this study, we investigate the level of implicit race information available to ML models and human experts and the implications of model-detectable differences in clinical notes. Our work makes three key contributions. First, we find that models can identify patient self-reported race from clinical notes even when the notes are stripped of explicit indicators of race. Second, we determine that human experts are not able to accurately predict patient race from the same redacted clinical notes. Finally, we demonstrate the potential harm of this implicit information in a simulation study, and show that models trained on these race-redacted clinical notes can still perpetuate existing biases in clinical treatment decisions.
I’ve always been interested in public health, so I’m excited to participate in this SuperUROP because it investigates whether racial biases exist in clinical notes and explores ways in which machine learning can be used to inform fairer clinical practice. Through this experience, I hope to gain a better understanding of topic models and natural language processing in general.