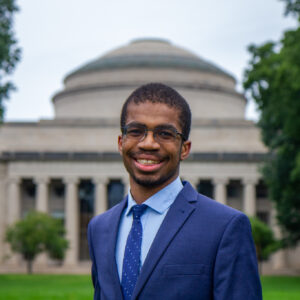
Maxim Noel Attiogbe
Eric and Wendy Schmidt Center Funded Research and Innovation Scholar
Enhancing Genetic Structural Variant Discovery with Deep Learning
2024–2025
Electrical Engineering and Computer Science
- AI for Healthcare and Life Sciences
Caroline Uhler
Accurate mapping of genomic structural variants (SVs) is crucial for advancing biomedical research and understanding genetic diversity. Deep learning is a promising approach for interpreting complex SV signatures in DNA sequencing data. Using data from the Human Genome SV Consortium, we will develop a convolutional neural network to detect SVs from short-read sequencing data, building on existing architectures for smaller mutations. We will then integrate the model into the Talkowski lab’s GATK-SV pipeline, creating a scalable, cloud-based workflow for structural variant filtering in large-scale genomic studies. This integration aims to enhance the accuracy and efficiency of identifying disease-causing genetic variants in research and clinical settings.
I am pursuing this project to gain experience combining research and engineering to develop a high-impact, production-ready healthcare application of machine learning. I aim to expand upon my ML research experience from CSAIL and SWE/ML industry experience from Microsoft and LinkedIn. This project will inform my ML research project choice for my MEng I plan to start in fall 2025 and my graduate school or industry decisions afterwards.