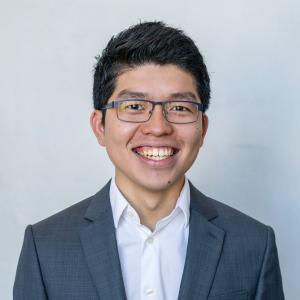
Loc Q Trinh
MIT EECS | Lincoln Laboratory Undergraduate Research and Innovation Scholar
Semi-Supervised Learning for Clinical Phenotyping
2017–2018
EECS
- Artificial Intelligence and Machine Learning
David Sontag
My project aims to learn the phenotype of a patient, consisting of hundreds of different clinical state variables based on routinely collected, unlabeled, and noisy clinical data available in Electronic Medical Records (EMRs). These state variables help describe the patient and provide answers to high-level questions, such as whether the patient has an infection, an altered mental state, or a history of alcoholism, which are not obvious or directly observable from the clinical records. Obtaining these labeled descriptions for a supervised learning algorithm is often expensive and requires extreme efforts from clinicians. We hope to be able to efficiently and accurately infer these phenotypes from unlabeled clinical data in a semi-supervised manner, incorporating specialist expertise as guidance.
Through this SuperUROP project, I want to gain more experience in health care research and make a positive contribution to my group. I have taken machine-learning courses and I want to expand on that knowledge with real-world applications, creating a social impact with my work. To me, even a 1 percent improvement in the field means a lot. I hope to publish a paper by the end of the SuperUROP project, if I have meaningful results to display.