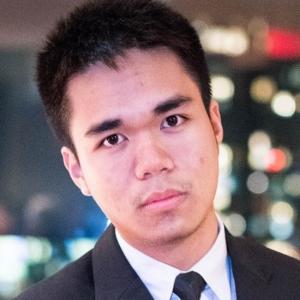
Kritkorn Karntikoon
MIT EECS | Lal Undergraduate Research and Innovation Scholar
Climate Networks and Ricci Curvature
2017–2018
EECS
- AI and Machine Learning
Stefanie Jegelka
We propose a method to reconstruct and analyze the global climate network via the geometric lens of Ricci curvature. The global climate can be seen as a large, evolving network between different grid points on the globe. Modern network analysis techniques are increasingly applied to understand this network by measuring mutual information and centrality of the network. However, existing techniques are often limited because of scalability and limited applicability to only unweighted networks, so we will combine Ricci curvature with the old method to solve the constraints. Ricci curvature is a geometric property (in some sense measuring shape) that can be discretized to (weighted) graphs via Ollivier-Ricci curvature and Forman-Ricci curvature. These graph curvatures have already been applied to social, brain and economic networks, and are shown to give insights into structural properties of these networks. Using Ricci curvature, we will perform a mathematical analysis to extract and observe the property of the climate network in more scalable and applicable way.
I am interested in this SuperUROP project because it is a combination of discrete mathematics, theoretical computer science, and machine learning. I think it will be enjoyable to use my knowledge in these fields to create a visualized result. I also hope that, by doing this project, I will be able to develop my skills and help solve real-life problems involving the global climate.