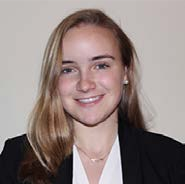
Katherine Pelton
MIT EECS | Nutanix Undergraduate Research and Innovation Scholar
Localizing Damage and Humanitarian Risk in Crowd-sourced Images and Text
2021–2022
EECS
- CS+HASS
Miho Mazereeuw
Over the past 50 years, the number of disasters has increased by a factor of five and caused on average US 202 million in losses daily. With the rate of climate disasters increasing, the efficiency of disaster response is becoming ever more important. Simultaneously, social media data is consistently increasing as a rich source of disaster information. We provide an approach to automatically localize damage and human risk in crowd-sourced images and text respectively. For images, we train a convolutional neural network and apply the Gradient-weighted Class Activation Mapping (Grad-CAM) to highlight areas of damage. For text, we apply a logistic regression and Local Interpretable Model-Agnostic Explanations (LIME) to highlight words indicative of human risk. Our method highlights key information in crowd-sourced data and acts as an alternative to expensive and inefficient human processing of disaster data.