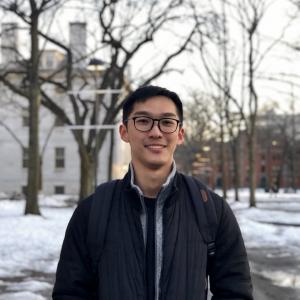
Justin K. Lim
MIT EECS | Hewlett Foundation Undergraduate Research and Innovation Scholar
Learning Optimal Treatment Policies for Chronic Disease
2019–2020
EECS
- Artificial Intelligence and Machine Learning
David Sontag
Chronic disease is a major public health problem in the developed world. These diseases tend to be managed over time, rather than cured outright, and many conditions are poorly understood. Our goal is to use large observational datasets (e.g., electronic medical records, health insurance claims) to learn optimal dynamic treatment policies for chronic diseases, such as multiple myeloma and congestive heart failure, and to personalize these policies based on individual patient characteristics and their response to treatment over time. We propose to develop methods to learn and evaluate such policies, including methods for checking the causal assumptions of models from epidemiology and reinforcement learning, as well as extending methods for the setting of a single action to a dynamic regime.
“Through SuperUROP, I would like to gain experience in clinical machine learning research. I have taken classes in machine learning and inference, such as 6.036 (Introduction to Machine Learning) and 6.438 (Algorithms for Inference), and want to apply my knowledge to solve problems with real-world impact. I hope to further my knowledge in causal inference, and I am excited to help advance our understanding of chronic diseases and how best to treat them.”