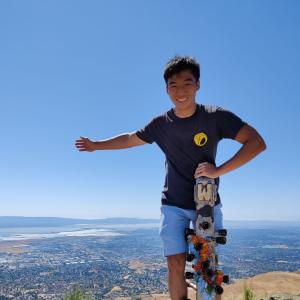
Julius Tao
MIT EECS | CS+HASS Undergraduate Research and Innovation Scholar
Variance in Training Neural Networks
2021–2022
EECS
- Artificial Intelligence and Machine Learning
- CS+HASS
Aleksander Madry
Even when we fix the architecture and dataset in machine learning experiments, we see significantly different results between models of different training runs. The focus of my research is estimating how different sources of randomness contribute to the variation of model predictions across experiments. Prior research has quantified the variance of accuracy or error on the test set, but I aim to measure the variance of each test data point.
Through SuperUROP, I hope to gain more authentic research experience. I’m prepared from learning PyTorch and OpenCV during internships, as well as statistics and algorithms from classes. I wish to learn firsthand the frustrations of failed experiments and hopefully the rush from success and its publishable results. I’m most excited about the many opportunities to work with and learn from people much more intelligent and experienced than me.