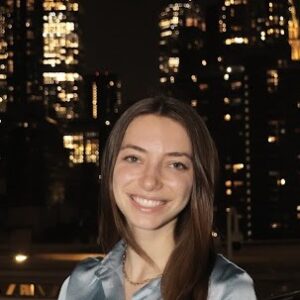
Julie Steele
MIT EECS | Nadar Foundation Undergraduate Research and Innovation Scholar
Unrestricted Adversarial Training
2024ā2025
Electrical Engineering and Computer Science
- Graphics and Vision
Nir N. Shavit
Currently all machine learning models are easily fooled by an adversarial perturbation to the input. Adversarial images are any images unanimously as one class to humans, yet classified as another by the image model. While the field has improved on adversarial robustness against small bounded perturbations, adversaries are not constrained. Our research applies, trains, and evaluates Binary Adversarial Training in the unrestricted adversarial setting. Binary Adversarial Training contrasts with regular adversarial training by penalizing entering an adversarial target-class instead of rewarding staying in the original image class. In addition, we extend Binary Adversarial Training to multiple classes. We evaluate with suites of gradient-based image attacks.
I love research because I love puzzling over hard problems. Iām excited to think creatively about how to solve this unsolved problem of training a robust image classifier, and to gain more hands-on experience training models and crafting research directions. In addition, I hope to improve my technical communication through SuperUROP. I hope research in adversarial robustness can get us closer to building trustworthy AI models.