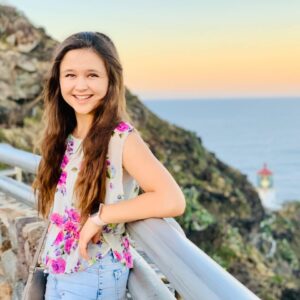
Julia Moseyko
MIT EECS | Hudson River Trading Undergraduate Research and Innovation Scholar
Constructing Human-Like Reinforcement Learning Policies Leveraging Model Uncertainty
2020–2021
EECS
- Artificial Intelligence and Machine Learning
Daniela L. Rus
In reinforcement learning constructs, it is important that agents perform well across diverse environments. Furthermore, rather than learning to execute specific narrow tasks, agents must efficiently learn generalizable skills leveraging human priors, such that these skills can be used to solve wide ranges of complex tasks. For example, in autonomous driving, an agent should learn fundamental driving skills, but adapt its behavior under different conditions (e.g., road, weather, speed). Therefore, the aim of this project is to use imitation-based approaches to guide reinforcement learning during training when the model is uncertain. The model is therefore encouraged to explore scenarios it might not encounter with pure IL, but it takes significantly less training episodes to converge.
I am excited to participate in SuperUROP to delve further into research and spearhead a project. I loved UROPing in the Distributed Robotics Laboratory the past two years, and I am thrilled and grateful to continue research with my mentor and supervisor. I am most excited about making progress towards more generalizable and practical reinforcement learning, and I hope to learn more about the current limitations and frontiers of the field.