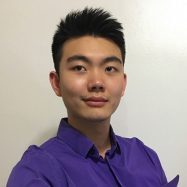
Jing Chao Lin
MIT EECS Keel Foundation Undergraduate Research and Innovation Scholar
Self Supervised Convolutional Neural Networks for Object Detection and Recognition
2016–2017
Electrical Engineering and Computer Science
- Robotics
Aude Oliva
Understanding and learning visual numeracy with attentional neural networks
Deep neural networks excel at a variety of visual tasks. Cutting edge networks combine convolutional and recurrent networks to extracts rich high dimensional image features find key sequential relationships from the input data. These networks are applied to difficult tasks like visual question answering (VQA). However current networks in VQA don’t have any attentional mechanisms which may boost performance by directing computations to more interesting image regions. We collect a novel dataset of human eye fixation positions on images shown after participants were asked visual questions about the image. We then train deep learning networks to fixate on informationally dense regions in input images evaluate our accuracy on the MSCOCO-VQA dataset and compare it with current benchmarks.
I took 6.869 and am taking 6.864 collected mobile eye tracking data in another UROP have worked with AWS and spent a summer at a computer vision startup building their deep learning framework. I hope to gain more intuition behind how neural networks function. I am really excited that the project draws inspiration from behavioral data and I’m curious to find out how that affects other properties of the network.