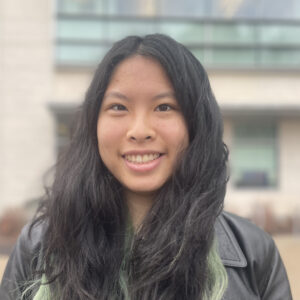
Jakin S. Ng
MIT EECS | Lincoln Laboratory Undergraduate Research and Innovation Scholar
Learned Initializers for Iterative PDE Solvers
2023-2024
Mathematics, Electrical Engineering and Computer Science
- AI and Machine Learning
Justin Solomon
Partial differential equations (PDEs) are ubiquitous throughout science, describing fundamental physical phenomena. Designing efficient and accurate numerical solvers is crucial but highly non-trivial. We propose using Fourier neural operators (FNOs), which learn the solution mapping for a family of PDEs, as learned initializers for iterative PDE solvers. By initializing close to the actual solution, the number of iterations required for the solver to converge is reduced, while achieving at least equivalent convergence and accuracy guarantees. Advantages include reduced computational cost for an entire family of PDEs, as well as equivalent accuracy to the original numerical solver.
During the SuperUROP program, I am looking forward to experiencing the full research process, as well as learning to communicate and present my results more effectively. I’m most excited to work on integrating ML and numerical methods to solve PDEs quickly and accurately.