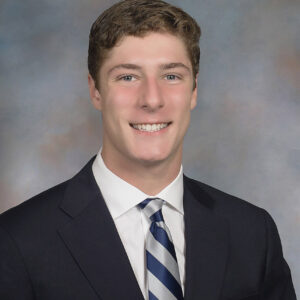
Jacob R. McCarran
MIT EECS | Undergraduate Research and Innovation Scholar
State Estimation and Control of Many-Body Quantum Systems Using Deep Learning Transformers
2024–2025
Electrical Engineering and Computer Science
- Quantum Computing, Communication, and Sensing
Dirk R. Englund
We investigate novel quantum control algorithms utilizing deep learning transformers within reinforcement learning (RL) frameworks for quantum control. Transformers excel at capturing correlations across multiple qubits and representing entanglement in large quantum systems. By employing transformers to dynamically adjust and optimize control parameters in real time, we aim to outperform existing techniques in preserving entanglement and coherence, advancing quantum computing technologies. We will compare how our transformer’s attention mechanism represents a quantum state compared to information theoretic bounds such as Bayesian estimators and belief propagation, demonstrating their effectiveness in heralded optical links of spin qubits within an RL framework.
I am excited to join Prof. Englund’s group for SuperUROP and learn more about using reinforcement learning to control quantum optics setups. I hope to further my knowledge in quantum engineering and use my previous UROP experience simulating Rydberg arrays to help build improved quantum control algorithms.