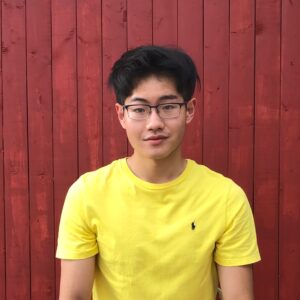
Howard N. Zhong
MIT EECS | Keel Foundation Undergraduate Research and Innovation Scholar
Investigating Race and Gender Biases in the NFT Market
2021–2022
Electrical Engineering and Computer Science
- Graphics and Vision
William T. Freeman
Non-Fungible Tokens (NFTs) are non-interchangeable assets, usually digital art, that are stored on the blockchain. The NFT market has skyrocketed since the start of 2021 and has become a critical element of the growing metaverse, which aims to connect people from all backgrounds in the virtual world. There is preliminary evidence raising diversity concerns by finding female and darker-skinned NFTs to be valued less than their male and lighter-skinned counterparts respectively, but these studies are not statistically rigorous and limited to only the CryptoPunks collection. We present the first study that tests the statistical significance of race and gender biases and scales to the comprehensive NFT market. We find evidence of systematic bias in race, but in contrast to preliminary studies in literature, we do not find evidence of gender bias. Our work introduces a new dataset to study nuanced socioeconomic effects in this emerging market, which is significant from a cultural standpoint to uphold fairness in the digital world.
I’ve enjoyed working in UROPs for the past 2 years and am looking forward to dedicate more energy into research with SuperUROP. I’m particularly excited to work on an interdisciplinary project that applies machine learning, statistics, and economics tools learned in classes to investigate diversity problems in the growing NFT space.