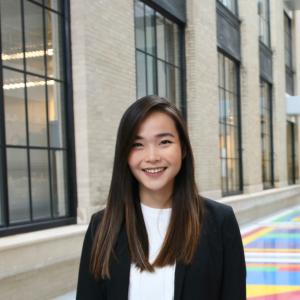
Fransisca Susan
MIT EECS | Keel Foundation Undergraduate Research and Innovation Scholar
What do Neural Machine Translation Models Infer about Multiple Semantics of Homonyms?
2017–2018
EECS
- Natural Language Processing & Speech
Jim Glass
Neural machine translation (NMT) has achieved state-of-the-art performances for various language pairs. However, little is known about what these models learn regarding the source and target language semantics in the training process. Our project develops a model to explore how accurate NMT models are at inferring the meaning of homonyms. We will investigate two NMT models: word-based vs. character-based. We will also explore how the depth of the encoding layer in a model and the identity of the target language impact the model’s ability to differentiate the multiple meanings of homonyms. We hope to learn what NMT models infer about the different semantics of homonyms as the necessary first step to improve the NMT models’ ability in translating sentences containing homonyms.
Through the SuperUROP program, I hope to gain valuable research experience and guidance and become more capable of working independently. I am excited about my project because I have always been curious to see how a machine can understand the words we speak. I hope to further my knowledge in machine learning and contribute to a meaningful area of research.