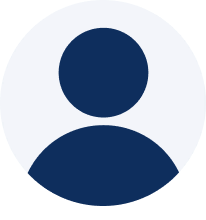
Eesha Banerjee
Undergraduate Research and Innovation Scholar
Developing Machine Learning Based Preconditioners for Solving the Dirac Equation in Lattice Quantum Chromodynamic Theory
2023–2024
Electrical Engineering and Computer Science
- Quantum
Phiala Shanahan
To explore physics beyond the Standard Model and explain, for instance, the nature of dark matter, we need to probe the limits of our understanding of fundamental physics, which is based on quantum field theories such as quantum chromodynamics (QCD): high precision and computationally expensive QCD calculations of physical observables are needed to provide predictions for experimental observations. Even lattice QCD calculations come with large computational costs. Dr. Shanahan’s lab has made great strides using ML tools to tackle this problem, by using normalizing flows to more efficiently sample lattice quantum field distributions to generate field configurations (Abbott et al 2022). Another computationally expensive side of the lattice QCD computational puzzle deals with calculations of physical observables over these configurations. My project uses neural network based preconditioners to increase the efficiency of these calculations by decreasing the iterations the Dirac solvers.