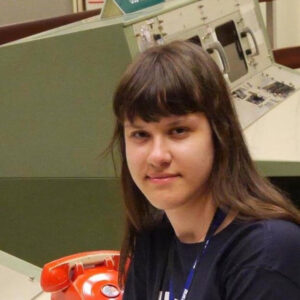
Diana Wofk
MIT EECS | Analog Devices Undergraduate Research and Innovation Scholar
Energy-Efficient Deep Neural Network for Depth Prediction
2017–2018
EECS
- Integrated Circuits and Systems
Vivienne Sze
Depth-sensing is a key aspect of navigational systems in autonomous robots. The ability to reconstruct a dense depth map of a scene from RGB imagery and sparse depth data is necessary for obstacle detection and motion planning. Since deep convolutional neural networks (DNNs) have achieved high accuracy rates in image classification and regression, recent work has focused on designing DNNs for depth prediction tasks. However, the high accuracy of DNNs comes at the cost of high computational complexity and energy consumption, and current designs are unsuitable for low-power applications in miniaturized robotics. This project seeks to address this gap by applying recently developed methodologies for estimating and improving the energy-efficiency of DNNs to an existing depth-prediction DNN.
I enjoyed courses in signal processing and digital system design and have developed an interest in multimedia applications. This project will allow me to learn more about vision deep learning and energy-efficient hardware architectures. I am excited to work on a long-term project that may lead to a publication. I am participating in SuperUROP to benefit from the rigor of the program and to improve my project and presentation skills.