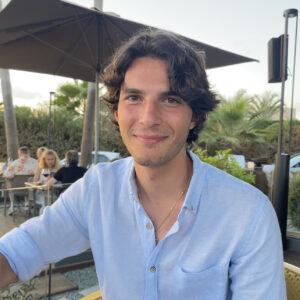
Charalampos (Charis) Georgiou
MIT EECS | Lincoln Laboratory Undergraduate Research and Innovation Scholar
Conformal Prediction for Optimization Informed Keypoint Placement
2024–2025
Electrical Engineering and Computer Science
- Graphics and Vision
Pulkit Agrawal
Luca Carlone
Accurate keypoint placement is crucial for robotics applications such as pose estimation and trajectory optimization. Current keypoint detectors, however, lack guarantees on output accuracy, leading to propagated errors in pose estimation algorithms. To address this, we propose training a diffusion model tailored to a specific category of objects to standardize keypoint selection. This approach aims to enhance pose accuracy across all objects within the category. We plan to integrate robust pose estimation algorithms directly into the training process using differentiable optimization layers. This will allow us to simultaneously optimize pose error and optimization tightness. Additionally, by utilizing conformal prediction, we can provide statistical guarantees on keypoint accuracy and predict their impact on pose estimation algorithms.
Through this SuperUROP, I aim to tap into my background in computer science and mathematics to come up with innovative solutions. I’m particularly eager to apply my skills to develop algorithms that improve robotic efficiency and precision. In general, I enjoy attempting problems that bridge multiple disciplines, and which benefit from closing the gap between theoretical knowledge and practical application!