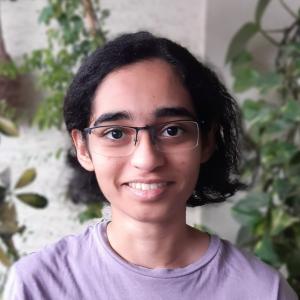
Aparna Ajit Gupte
MIT EECS | Keel Foundation Undergraduate Research and Innovation Scholar
The Cryptographic Hardness of Learning
2021–2022
EECS
- Theory of Computer Systems
Vinod Vaikuntanathan
Despite the empirical success of machine learning algorithms, there are still gaps between the algorithmic upper bounds and hardness lower bounds. There have been several results proving the hardness of learning problems assuming the security of common cryptosystems. These results are often more meaningful, because the average-case assumptions in cryptography translate to lower bounds for learning problems in more realistic settings, where the inputs are drawn from some specific distribution. This project aims to further explore the connections between problems from lattice-based cryptography and the hardness of learning. In particular, we are interested in the hardness of learning Gaussian mixtures, and the hardness of learning neural networks.
I’m excited to participate in the SuperUROP program this year, and look forward to spending time thinking about problems in the space of hardness of learning. Through this SuperUROP, I hope to learn more about lattice-based cryptography as well as learning theory, and work towards becoming a better researcher.