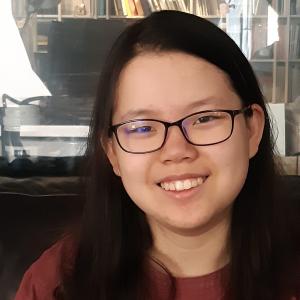
Andrea Jessica Jaba
MIT EECS | Himawan Undergraduate Research and Innovation Scholar
Improving the Explainability of CNN-Based Image Classifiers for Scene Recognition
2019–2020
EECS
- Artificial Intelligence & Machine Learning
Lalana S. Kagal
Since disease diagnosis is often a life-critical and liability-sensitive application, these systems require explanations to ensure trustworthiness and, in case of failures, for the purpose of assigning liability and determining corrective action. We propose to extend nascent techniques for extracting rules from deep neural networks, leveraging semantic information and visualization techniques, in order to generate understandable explanations of network decisions and behavior.
“I am participating in SuperUROP because I want to learn more about explainability in machine learning. I have taken machine learning classes in the past mostly concerned with how to create and refine a good model but I think knowing how to explain the results of a model is just as important. I am excited to learn more about the topic, especially in the field of medicine, and I would hope to be able to publish a paper by the end of the year.”