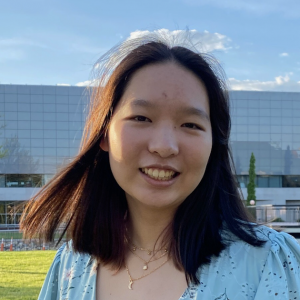
Amelia Hu
MIT EECS | Philips Undergraduate Research and Innovation Scholar
A Recurrent Network Approach to G-Computation for Sepsis Treatment Outcome Prediction under Dynamic Treatment Strategies in the ICUs
2022–2023
Electrical Engineering and Computer Science
- AI and Machine Learning
Roger Mark
In clinical settings, doctors often have to quickly make choices between various treatment options for their patient depending on the patient’s current state. G-Net is a recurrent neural network that predicts the probability of an adverse outcome if a patient was given a specific treatment plan over a period of time. We plan on examining G-Net’s applications to analyze treatment options for sepsis (when the body has an extreme response to infection). Treatment for sepsis involves administering fluids and/or vasopressors and our goal is to use G-Net to model the optimal balance of fluids and vasopressors given patient data. In particular, we will apply G-Net to estimate effects of alternative sepsis treatment strategies using real-world data from the intensive care units.
I am participating in SuperUROP so that I can gain more experience in academic research. I have taken machine learning courses that will prepare me for my project. In particular, I hope to learn how to apply the ML techniques from class to research and am excited to be working on a project with applications in medicine.